Mastering Data Annotation: The Essential Guide to Annotate Pictures
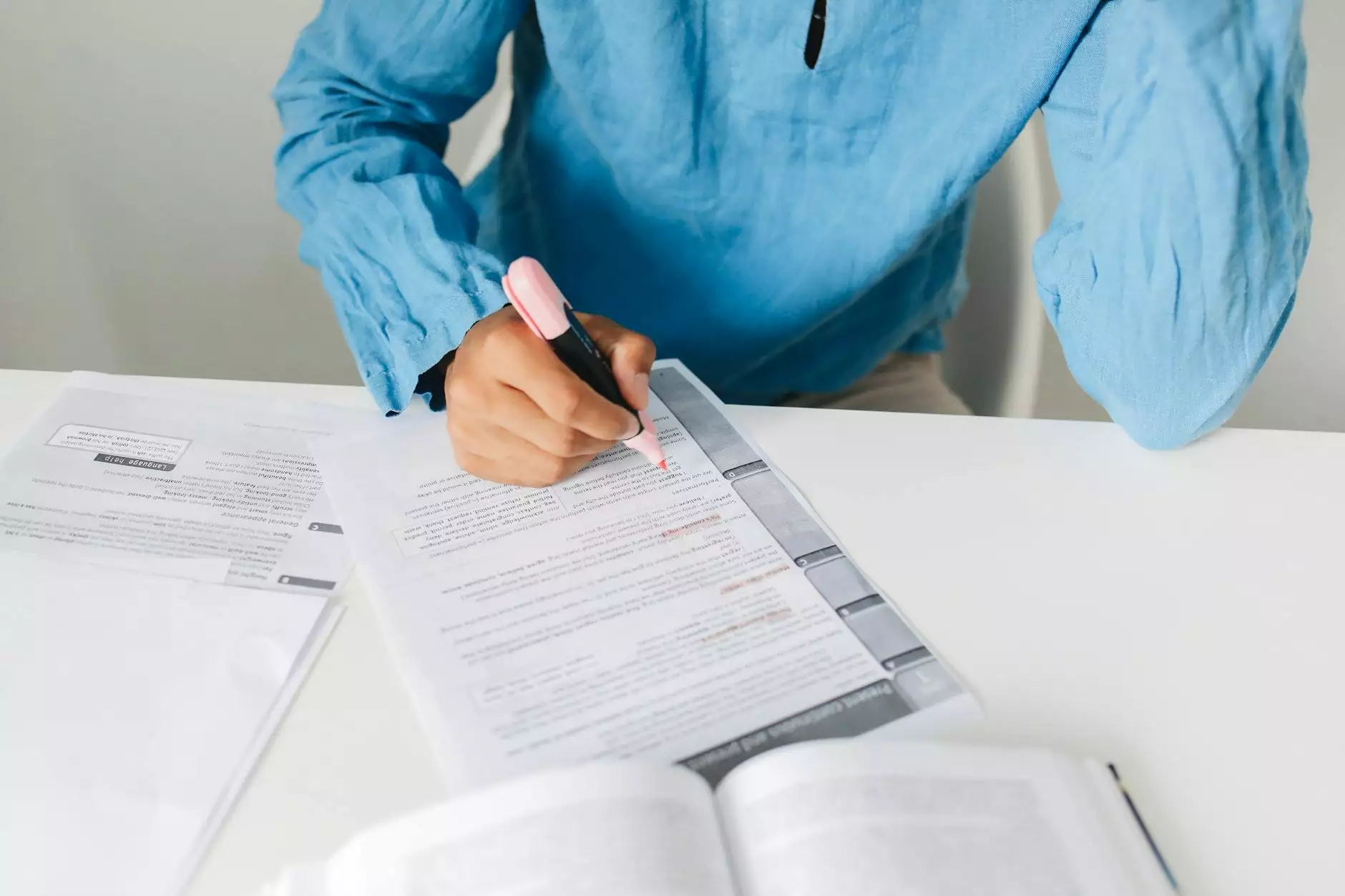
In today's data-driven world, businesses are increasingly reliant on artificial intelligence (AI) and machine learning (ML) technologies to innovate and stay competitive. One critical aspect of training these advanced systems is the ability to annotate pictures effectively. This comprehensive guide delves into the importance of data annotation, explores various tools and platforms available, and provides practical strategies to enhance your workflow.
Understanding Data Annotation
Data annotation refers to the process of labeling or tagging data to provide context for machine learning algorithms. This process enables AI systems to learn from data by identifying and classifying patterns and features within images. As a business owner or decision-maker, understanding the significance of data annotation can vastly improve your operations and outcomes.
Why is Data Annotation Important?
- Enhanced Accuracy: Properly annotated data leads to more accurate predictions and insights from AI models.
- Improved Training: A well-annotated dataset serves as a solid groundwork for training machine learning models, ensuring they learn effectively.
- Time Savings: Efficient data annotation can significantly reduce the time taken for model training cycles.
- Scalability: As your business grows and data needs expand, scalable annotation solutions will keep pace with your requirements.
Types of Data Annotation Techniques
There are various methods available for annotating pictures, each suited for different types of AI applications. Here are some common techniques:
1. Image Classification
This involves categorizing images into predefined classes. For instance, if you're developing a facial recognition system, you can classify images as 'smiling' or 'not smiling'. This technique is essential for sorting and managing large datasets.
2. Object Detection
Object detection goes a step further, not only identifying the class of objects in an image but also locating them within the frame. This is crucial for applications like autonomous vehicles, where identifying and pinpointing obstacles is paramount.
3. Image Segmentation
Image segmentation divides an image into meaningful segments or regions. Each pixel of an image is assigned a label, enhancing the granularity of analysis. This technique is often used in medical imaging to identify particular structures within scans.
4. Keypoint Annotation
This technique focuses on marking specific points of interest within an image. For example, in gesture recognition systems, keypoints on a human body can be annotated to train models effectively.
Choosing the Right Data Annotation Tool
The effectiveness of your data annotation efforts largely depends on selecting the right tools and platforms. Here are key considerations when evaluating data annotation solutions:
1. Usability
A good annotation tool should be intuitive and easy to navigate. This ensures that your team can start annotating data quickly without extensive training.
2. Customization
Look for platforms that allow customization to align with your specific needs. The ability to create unique annotation labels or workflows can greatly enhance efficiency.
3. Scalability
Select tools that can scale with your growing data needs. As your dataset expands, your annotation solution should be capable of handling increased volumes without a drop in performance.
4. Collaborative Features
Collaboration tools are essential for teams working together on data annotation projects. Features such as real-time commenting, sharing, and version control can significantly boost productivity.
Exploring KeyLabs.ai: A Premier Data Annotation Platform
When it comes to annotating pictures, KeyLabs.ai stands out as a premier data annotation platform. It offers a suite of powerful features designed to streamline the annotation process:
1. Advanced Toolset
KeyLabs.ai provides a comprehensive set of tools for various annotation tasks including bounding boxes, polygons, and semantic segmentation. This flexibility allows businesses to tailor their approach based on specific project requirements.
2. AI-Assisted Annotation
Utilizing cutting-edge technology, KeyLabs.ai incorporates AI to assist in the annotation process. Machine learning algorithms can suggest labels and speed up the manual annotation process, drastically reducing time and effort.
3. Quality Control Measures
The platform implements strict quality control measures to ensure high accuracy in annotations. Through automated checks and manual reviews, businesses can trust that their data is correctly annotated, leading to superior AI outcomes.
4. Secure Data Handling
KeyLabs.ai prioritizes data security and confidentiality, adhering to industry standards. This assurance is essential for businesses handling sensitive information.
Best Practices for Effective Data Annotation
Implementing best practices can significantly improve your data annotation efforts. Here are some recommended strategies:
1. Define Clear Annotation Guidelines
Establishing clear and comprehensive guidelines is crucial for consistency in the annotation process. These should detail how to annotate various elements, ensuring every team member is aligned in their approach.
2. Invest in Training
Providing training to annotators enhances their understanding of the task at hand. Regular training sessions can also help improve the overall quality of the annotations.
3. Utilize Iterative Feedback
Implement a system for providing continuous feedback to annotators. An iterative approach allows for adjustments and improvements over time, fostering an environment of constant learning.
4. Monitor Progress and Performance
Regularly assess the performance of your data annotation efforts. Using metrics such as accuracy rates and time taken per annotation helps identify areas for improvement.
The Future of Data Annotation
As AI technologies evolve, the importance of efficient and effective data annotation will only increase. Businesses invested in incorporating AI and ML will benefit from high-quality annotated data. Looking ahead, here are some trends to watch:
1. Automation and AI-Driven Annotation
The future will see a greater emphasis on automation in data annotation. Tools that leverage AI to automate tedious tasks will significantly improve efficiency.
2. Integration with Machine Learning Workflows
Data annotation platforms will increasingly integrate directly with machine learning workflows, allowing for seamless transitions from data collection to model training.
3. Enhanced Collaboration Tools
As teams become more geographically dispersed, the need for robust collaborative features within annotation platforms will become more pronounced. Real-time editing and feedback will enhance remote teams' productivity.
Conclusion
In conclusion, mastering the art of data annotation is vital for businesses seeking to harness the power of AI. By understanding the significance of effectively annotating pictures, selecting the right tools, and implementing best practices, companies can enhance their data quality and achieve better outcomes. Embrace the future of data annotation with solutions like KeyLabs.ai, and transform your data into a valuable asset.